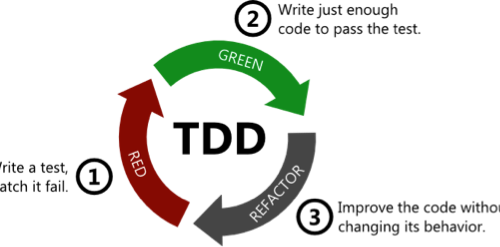
It Changed My Life – Book Five
XP Explained ruined software engineering for me. Continue reading
XP Explained ruined software engineering for me. Continue reading
Diversity is good for software companies. It helps us make better products. Continue reading
90% of science fiction is crap. 90% of everything is crap. Continue reading
I was wondering what songs are about. Most of them are about love of course but what about the other ones? Terry Gross had a dude on the other day who wrote songs about a bomb that went off his … Continue reading
Python. It’s not quite Ruby. Continue reading
Me: Do you know ruby or java? Other guy: No. Php and Javascript. Me: How about you? C#, maybe? Third guy: No. Just Perl. Me: Anyone know Python? All: No. Me: OK, me neither. Let’s do Python, then.
I was bored with my wordpress theme and Stu’s fresh look made me decide it was time for a refresh. This is my third theme and I wanted to go right back to basics this time rather than copy an … Continue reading
The Hacker’s Diet gives us two tools for bringing wayward eating under control. The first one is the little red dot that says your weight is trending upwards. As you can see, i’ve been ignoring the red dots for too … Continue reading
Rhapsody’s service has been spotty recently so I thought I’d try Spotify to see if it is all it is cracked up to be. And the verdict is… …it’s OK. But like every other music app I have ever tried … Continue reading
I’ve been following John Walker’s Hacker’s Diet for about nine months now. I love the simplicity of it. “Anyone can control their weight. It’s a simple matter of balancing calories.” – John Walker Mr Walker’s book is a fun, simple … Continue reading